Harnessing AI to Create Targeted Customer Cohorts
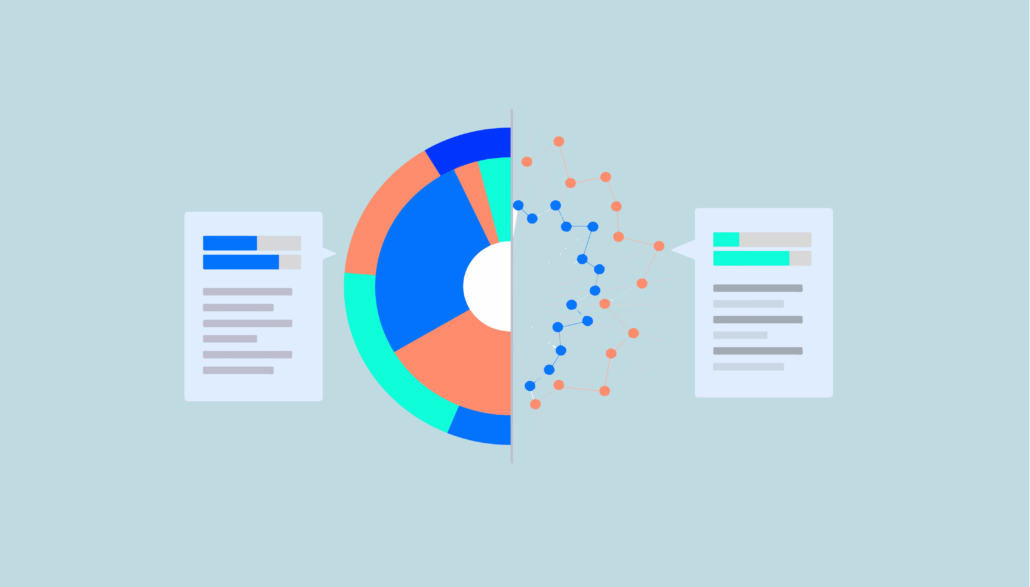
Over the last year, we leveraged artificial intelligence to analyze five years’ worth of customer transaction data to determine if there were distinct groups, or cohorts, within our customer base. The goal was clear: identify patterns that could inform more strategic and effective marketing efforts. The results exceeded our expectations.
The AI-Driven Analysis
Rather than relying on conventional segmentation methods, we let AI take the lead. By analyzing historical purchase data, AI surfaced four primary cohorts based on transactional behavior:
-
Independently Transacting: Customers who placed orders in more than two consecutive busy seasons.
-
Occasionally Transacting: Customers with transactions in fewer than two consecutive busy seasons.
-
Dormant: Customers who had not placed an order in the last 12 months.
-
Account Created: Customers who had an account but had never transacted.
This initial segmentation provided a foundation, but we wanted to take it further. By layering in school type (college, university, community college, trade school), public vs. private status, and full-time enrollment, we started seeing additional trends emerge.
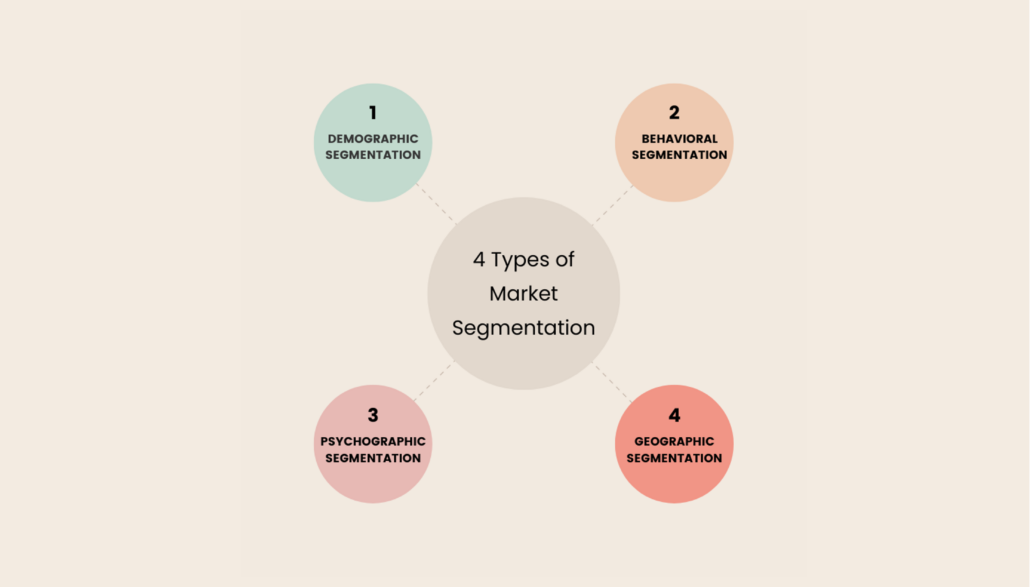
Uncovering Patterns with AI
Once we grouped customers by both behavior and institutional attributes, AI helped us identify fascinating correlations. Certain school types over-indexed in specific cohorts. For example, community colleges had a higher percentage of Dormant accounts, while private universities had a greater share of Independently Transacting customers. These insights allowed us to anticipate behaviors and understand how to re-engage segments more effectively.
Beyond school segmentation, AI identified purchase behavior nuances that had previously gone unnoticed. We saw that some trade schools had peak purchasing times outside of the traditional academic calendar, while larger public universities had a more consistent, predictable cycle. This level of insight allowed us to craft more strategic, data-driven engagement strategies.
Customized Marketing Plans: The Execution
With these AI-driven insights, we created hyper-targeted marketing strategies for each cohort. Rather than deploying generic campaigns, we tailored messaging to match the customer’s behavioral patterns and institutional attributes:
-
For Occasionally Transacting customers, we focused on emphasizing ease of ordering and exclusive promotions during their peak purchasing windows.
-
For Dormant customers, we introduced urgency-driven messaging combined with curated product recommendations based on previous purchases.
-
For Account Created customers, we designed onboarding campaigns that guided them through their first purchase, addressing potential friction points.
The Impact: Measurable Revenue Growth
The results were undeniable. By implementing AI-driven segmentation and marketing automation, we saw a 50% increase in revenue from the Occasionally Transacting and Dormant cohorts. This approach not only improved engagement but also enhanced long-term customer retention.
Key Takeaways: AI in Customer Segmentation
-
Let AI do the heavy lifting: Traditional segmentation is useful, but AI can uncover patterns that might otherwise be missed.
-
Behavioral data is just the start: Combining transactional data with institutional characteristics unlocks deeper insights.
-
Personalization drives results: Customers respond to messaging that aligns with their habits and preferences.
-
Continuous learning is essential: AI-driven segmentation should be an ongoing process, adapting as customer behaviors evolve.
As we continue refining our AI-driven approach, the next frontier is predictive analytics—forecasting customer behavior before it happens and proactively engaging them. If our latest results are any indication, the future of AI-powered customer segmentation is bright, and the potential for revenue growth is even greater.